SPEAKERS
Keynote
Mihaela van der Schaar
University of Cambridge
John Humphrey Plummer Professor of Machine Learning, AI, and Medicine
Title
Pushing Medical Frontiers: AI-Driven Breakthroughs in Medicine
This presentation explores the transformative impacts of machine learning on personalized medicine and clinical trials, highlighting advancements in personalized screening, diagnosis, treatments, and monitoring. I will delve into automated machine learning for risk prediction and screening, causal effect inference for understanding treatment impacts, ODE and PDE discovery from data for pharmacology, time-series forecasting for patient monitoring, and continuous time control for optimizing interventions. By integrating these methods, we are not only enhancing the precision of medical care but also revolutionizing the efficiency of clinical trials. The talk will showcase real-world examples of how AI can redefine the entire healthcare landscape, providing insights into the current applications, challenges, and future directions of AI in medicine. Come discuss how AI can make these transformations in healthcare and join forces with us in this endeavour!
Mihaela van der Schaar is the John Humphrey Plummer Professor of Machine Learning, Artificial Intelligence and Medicine at the University of Cambridge and a Fellow at The Alan Turing Institute in London. In addition to leading the van der Schaar Lab, Mihaela is founder and director of the Cambridge Centre for AI in Medicine (CCAIM).
Mihaela was elected IEEE Fellow in 2009. She has received numerous awards, including the Oon Prize on Preventative Medicine from the University of Cambridge (2018), a National Science Foundation CAREER Award (2004), 3 IBM Faculty Awards, the IBM Exploratory Stream Analytics Innovation Award, the Philips Make a Difference Award and several best paper awards, including the IEEE Darlington Award.
Mihaela is personally credited as inventor on 35 USA patents (the majority of which are listed here), many of which are still frequently cited and adopted in standards. She has made over 45 contributions to international standards for which she received 3 ISO Awards. In 2019, a Nesta report determined that Mihaela was the most-cited female AI researcher in the U.K.
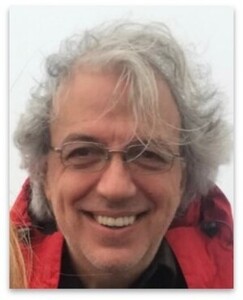
Alessandro Doria
Harvard Medical School and Joslin Diabetes Center
Professor of Medicine and Senior Investigator
Title
Leveraging genetics for AI-based personalized medicine of common multifactorial disorders
During the past two decades, the completion of the Human Genome Project and technological advancements have allowed unprecedented progress in our ability to study the human genome. This has led to the identification of thousands of genetic variants associated with common multifactorial disorders such as cardiovascular disease, diabetes, and cancer. Artificial intelligence offers the opportunity to integrate this genetic information with data concerning environmental exposures, previous medical history, and other clinical features in order to identify individuals at high risk of these disorders and develop preventive and therapeutic programs tailored to their individual characteristics. In this talk, I will use diabetes and other cardio-metabolic disorders as examples to illustrate how this personalized medicine approach can revolutionize health care by enhancing the effectiveness of preventive interventions and treatments while decreasing their adverse effects and financial costs. I will also review the challenges of implementing personalized algorithms in clinical practice and contrast those with the potential public health rewards that make this goal worth pursuing.
Dr. Doria is a Senior Investigator and Head of the Section on Genetics and Epidemiology at the Joslin Diabetes Center in Boston. He is also a Professor of Medicine at Harvard Medical School and a Professor in the Department of Epidemiology at the Harvard T.H. Chan School of Public Health. He received his medical degree and doctorate in endocrinology and metabolism from the University of Padua in Italy, and his MPH from the Harvard School of Public Health. Dr. Doria’s research is aimed at identifying genetic factors predisposing to the cardiovascular complications of diabetes and using this knowledge to find novel approaches to tackle this health problem. He is also active in the search for new interventions to prevent progression of diabetic kidney disease, serving as the founding Co-chair of the PERL (Preventing Early Renal Loss in type 1 diabetes) consortium. Dr. Doria recently served as an Associate Editor of the American Diabetes Association’s journal, Diabetes, and is a dedicated mentor to young researchers around the world in the fields of epidemiology, genetics, and diabetic complications. He was the recipient of the 2018 Edwin Bierman Award from the American Diabetes Association for outstanding contributions in the field of macrovascular complications of diabetes.

Sebastien Ourselin
AI Centre for Value Based Healthcare, Kings College London
Professor of Healthcare Engineering
Title
Learning across 16M patients: platforms and applications in acute care
The London AI Centre partner hospitals provide care to a quarter of the UK’s population. King’s College London, alongside three additional London universities, are building an AI and big-data infrastructure across 10 hospital Trusts to deliver the vision of AI-enabled care. The infrastructure, built with privacy in mind, will enable all clinical data (i.e. imaging, genetics, blood, pharmacy, interventions, medical decisions, costs) to be used for research, clinical and operational purposes, allowing algorithms to be trained on clinical data without data ever leaving the hospital. In order to achieve this, we are building large computing infrastructure within each hospital, a centralised orchestration system to enable federated learning across multiple hospitals, and a clinically-integrated AI deployment infrastructure to enable the translation of AI technologies into the real world. To demonstrate the value of this platform, the talk will introduce a large-scale research programme in Acute Neurology, built and deployed around this platform. This programme of research demonstrates how to make sense and extract value from real-world Neurological and Neuroradiological clinical data using advanced AI models, to enable the creation of new clinical tools that inform, augment and improve the treatment of patients with acute neurological conditions.
Professor Sebastien Ourselin FREng FMedSci, is the Head of the School of Biomedical Engineering & Imaging Sciences at King’s College London. He is also the Director of the Wellcome/EPSRC Centre for Medical Engineering and Deputy Director of the London Medical Imaging and Artificial Intelligence Centre for Value Based Healthcare. He has over 25 years of experience within academia and research organisations across three countries. Alongside Guy’s & St Thomas’ NHS Foundation Trust, he is leading the establishment of a MedTech Hub, located at St Thomas’ campus. The vision for the Hub is to create a unique ecosystem, enabling academia, industry and the NHS to work in synergy and develop health technologies (including medical devices), workforce and operational improvements that will be of global significance. He has significant experience in translating and commercialising healthcare technology and in MedTech entrepreneurship
Invited
Yael Ophir
HealthIL
Executive Director | HealthIL
Title
From Hype to Ripe: Implementing AI in Health Systems
Artificial Intelligence (AI) has emerged as a transformative force in healthcare, promising to revolutionize patient care, diagnostics, and operational efficiency within health systems. However, the journey from the initial hype surrounding AI to its successful implementation in health systems is a complex and multifaceted process. This session will delve into the critical aspects of transitioning from AI in healthcare as a speculative concept to a ripe, real-world application. It highlights the challenges and opportunities encountered during the implementation phase, discusses the key considerations for adopting AI technologies in health systems, and emphasizes the importance of a holistic approach that encompasses technological, ethical, and regulatory dimensions. By examining case studies and best practices from Israeli Health Organizations, this session will explore the evolving landscape of AI in healthcare, shedding light on how health systems can leverage AI’s potential to enhance patient outcomes, streamline processes, and achieve a mature, sustainable integration of AI technologies. The shift from hype to ripe represents a pivotal moment in healthcare, where the promise of AI can be translated into tangible benefits, ultimately improving the quality and accessibility of healthcare services.
Yael Ophir obtained her M.Sc. in Biomedical Sciences, summa cum laude, from the Hebrew University specializing in immunology and cancer research. Yael has intensive multidisciplinary experienced in creating value and integration between health organizations, tech companies and policy makers; Yael is also an expert of innovation management and training specifically in healthcare, as well as practical implementation of tech as part of organizational workflow.
Yael is the Executive Director of HealthIL, the leading healthcare innovation ecosystem in Israel, actively supporting transformation and innovation in healthcare systems, both in Israel and globally. HealthIL’s mission is to bridge the gap between healthcare systems, technology, and startups by building innovation infrastructures, addressing regulatory hurdles, and deeply understanding the challenges and opportunities of healthcare systems and technological solutions. Currently, HealthIL is supporting 65 Israeli health organizations in innovation management, and dozens worldwide; connecting them to +1,000 tech solutions, and driving more than 900 pilots and collaborations. HealthIL is an independent non-profit, supported by the Israeli Ministry of Economy, the Israeli Ministry of Health, the Israeli National Digitalization Agency, the Israel Innovation Authority, and the Israeli Innovation Institute.
Tianxi Cai
Harvard Medical School
Professor of Biomedical Informatics
Title
Crowdsourcing with Multi-institutional EHR to Improve Reliability of Real World Evidence – Opportunities and Challenges
The wide adoption of electronic health records (EHR) systems has led to the availability of large clinical datasets available for discovery research. EHR data, linked with bio- repository, is a valuable new source for deriving real-word, data-driven prediction models of disease risk and progression. Yet, they also bring analytical difficulties especially when aiming to leverage multi-institutional EHR data. Synthesizing information across healthcare systems is challenging due to heterogeneity and privacy. Statistical challenges also arise due to high dimensionality in the feature space. In this talk, I’ll discuss analytical approaches for mining EHR data to improve the reliability and generalizability of real world evidence generated from the analyses. These methods will be illustrated using EHR data from Mass General Brigham and Veteran Health Administration.
Tianxi Cai, PhD (Biostatistics), Professor of Biomedical Informatics (HMS) and Biostatistics (HSPH) and John Rock Professor of Population and Translational Data Science, Harvard University. Dr. Cai co-directs the VERITY Bioinformatics Core at Brigham and Women’s Hospital and an Applied Bioinformatics Core at Veteran Health Administration. She is the founding director of the Translational Data Science Center for a Learning Health System at HMS and HSPH. She also directs the Big Data Analytics Core at Harvard Medical School, providing statistical and biomedical informatics support to both Harvard research community and external research groups including VA and industry. Dr. Cai’s research team has successfully developed statistical and informatics tools for analyzing complex big biomedical data from large scale studies including electronic health records, cohort studies, disease registries, genomic studies, and randomized clinical trials.
Manlio De Domenico
University of Padua
Associate Professor of Applied Physics
Title
Complexity in health and disease: a network perspective to personalized medicine
As we navigate the intricate landscape of modern healthcare, it becomes evident that understanding health and disease is not a linear endeavor but rather a complex, multifaceted challenge. I will discuss the integration of complexity science and network theory as a framework for advancing the domain of personalized medicine, specifically by means of the rising paradigm known as “network medicine”. Drawing on cutting-edge research and methodologies at the crossroad of statistical physics, systems biology and computer science, we will unpack the complexity inherent in biological systems and how it correlates with states of health and disease.
The talk aims to bridge the gap between the promise of personalized medicine and its effective implementation, stressing the necessity for a multi-disciplinary perspective that reconciles in silico, in vitro and in vivo research.
Manlio De Domenico is Associate Professor of Physics at the Department of Physics and Astronomy “Galileo Galilei”, University of Padua (Italy) where he also directs the Complex Multilayer Networks Lab. He is a member of the Scientific Board of the Padua Neuroscience Center and Program Director of the Padua Center for Network Medicine. He is the national coordinator of the Italian Chapter of the Complex Systems Society and founding Director of the Mediterranean School of Complex Networks.
De Domenico is interested in a variety of complex systems, with works from human mobility and infectious disease spreading, to protein-protein interactions and connectomics. His research focuses on collective phenomena emerging from natural and artificial interdependent systems, with contributions to multiscale modeling and analysis of multilayer networks, their structure, dynamics, information capacity and resilience to shocks, finding applications in systems biology, systems medicine and computational epidemiology.
For his work he received the Young Scientist Award for Socio- and Econophysics from the German Physical Society (2020), the IUPAP Young Scientist Award on Statistical Physics from the IUPAP-C3 (2019), the USERN Prize in Formal Sciences from USERN (2017) and the Junior Scientific Award from the Complex Systems Society (2016).
He has published more than 160 scientific papers on peer-reviewed journals, attracting 20000+ citations (Sep 2023, Google Scholar).

Jens Rittscher
Oxford University
Professor of Engineering Science
Title
Beyond genomics – AI as an enabler for next-generation pathology
Alongside digital pathology, artificial intelligence is one of the key enablers to revolutionise pathology. It is the convergence of different imaging technologies and AI that will change the way pathologists work and potentially make pathology the hub for precision medicine. This talk will highlight recent work that aims to improve the accuracy of diagnosis and predict a patient’s response to therapy.
In the context of myeloproliferative neoplasms (MPN), a group of rare disorders of the bone marrow, we demonstrate that quantitative image analysis can improve the accuracy of diagnosis. The terminology used with the current classification scheme for MPN remains subjective, qualitative, and variably reproducible. In contrast to novel technologies for the detection of genetic aberrations in blood cancers, haematopathologists still summarise highly complicated tissue features as text-based reports. Our results demonstrate that a more quantitative approach allows defining disease subtypes and quantifying response to disease-modifying treatments.
Building on our work on predicting molecular subtypes of colorectal cancer from tissue morphology we present an approach of predicting a patient’s response to therapy. We use the question of predicting response to radiotherapy in colorectal cancer patients as an exemplar for developing prediction models that do provide such contextual information and therefore can effectively support clinical decision making. By jointly predicting a patient’s response to radiotherapy, the presence of a bespoke molecular subtype, and the epithelial tissue map from morphological features extracted from standard H&E slides, we provide a comprehensive clinically relevant assessment of a biopsy.
Jens Rittscher is Professor of Engineering Science at the University of Oxford with his appointment held jointly between the Institute of Biomedical Engineering and the Nuffield Department of Medicine. He is a group leader at the Big Data Institute and is affiliated with the Ludwig Institute of Cancer Research and the Wellcome Centre as an adjunct member. Previously, he was a senior research scientist and manager at GE Global Research (Niskyauna, NY, USA). His research interests lie in enabling biomedical imaging through the development of new algorithms and novel computational platforms, with a current focus to improve mechanistic understanding of cancer and patient care through quantitative analysis of image data. He is a co-director of the Oxford EPSRC Centre for Doctoral Training in Health Data Science. Presently, he serves on the executive committee of the Medical Image Analysis and the editorial board of Biological Imaging. In 2019 he co-founded the Oxford University Spinout company Ground Truth Labs.
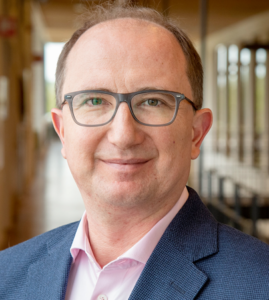
Matej Orešič
School of Medical Sciences, Örebro University, Örebro, Sweden
Professor of Medicine, with Specialization in Systems Medicine
Title
Exposomics approach as a tool to predict and prevent the disease
The exposome concept denotes “the totality of human environmental exposures from conception onwards, complementing the genome”. The exposome includes lifetime exposure, combining exogenous chemicals with genetic and other external factors that generate further molecular products inside the body and thereby may present threats to human health. In this context, environmental factors comprise the full suite of general external (e.g., socioeconomic status, education, climate), specific external (e.g., environmental pollutants, diet, drugs, occupational and internal (e.g., metabolism, gut microbiome, immune system status) exposures.
The origins of many non-communicable diseases occur often as a result of complex interactions of environmental and genetic factors. Thus, use of the ‘exposomics’ approach is important if one is to develop new strategies for disease prediction and prevention, and to implement personalized medicine. Key challenge of exposome research is vast amount of heterogeneous and multi-modal data that need to be integrated, analyzed, and interpreted. Thus, use of machine learning and AI in this context is becoming a necessity.
Here, recent and ongoing studies will be presented that aim to elucidate the link between the exposures to harmful chemicals and metabolic health. For example, growing evidence suggests that exposure to endocrine disrupting chemicals such as per- and polyfluoroalkyl substances (PFAS) interfere with host metabolism, however, the underlying mechanisms are poorly understood. In our research, we found that chemical exposures are linked with non-alcoholic fatty liver disease, obesity, and insulin resistance, with the impact being mediated by specific groups of metabolites such as steroids and microbially regulated secondary bile acids.
Matej Orešič holds a PhD in biophysics from Cornell University (1999; Ithaca, NY, USA). He is professor of medicine, with specialization in systems medicine at Örebro University (Sweden) and a group leader in systems medicine at the University of Turku (Finland). Prof. Orešič’s main research areas include exposomics and metabolomics applications in biomedical research and systems medicine. He is particularly interested in the identification of environmental exposures (exposome) and disease processes associated with different metabolic phenotypes and the underlying mechanisms linking these processes with the development of specific disorders or their co-morbidities. Prof. Orešič also initiated the popular MZmine open-source project, which led to the development and release of popular software for metabolomics data processing. As of 2016, he was made a Lifetime Honorary Fellow of the Metabolomics Society. Prof. Orešič currently serves as member of the Board of Directors of the Metabolomics Society and is one of the founders of the Nordic Metabolomics Society, previously serving as its chair of the board. In 2019, he co-chaired the 1st Gordon Research Conference on ‘Metabolomics and Human Health’ (Ventura, CA, USA). Previously, he also chaired the Keystone Symposium on Systems Biology of Lipid Metabolism (2015; Breckenridge, CO, USA).
Fabio Vandin
University of Padova
Full Professor of Computer Engineering
Title
AI in Cancer Genomics: Challenges and Future Directions
Sequencing technologies now allow measuring different features (e.g., somatic alterations) of a cancer genome at an outstanding level of detail for an unprecedented number of tumors. The resulting datasets provide an exceptional opportunity to gain insight into cancer development and progression by identifying patterns of alterations across different tumors. However, the identification of reliable patterns poses severe challenges, due to complex interactions among such features and the high dimensionality of the problem. In this talk I will present recently developed AI methods to identify reliable and significant patterns from measurements of a large collection of tumors, and highlight some of the current and future challenges in the use of AI in cancer genomics.
Fabio Vandin received the Laurea Triennale degree (summa cum laude, 2004) and the Laurea Specialistica degree (summa cum laude, 2006) in Computer Engineering from the University of Padova, Italy. He received the Ph.D. (2010) in Information Engineering from the University of Padova, Italy. He has been a postdoctoral researcher at Brown University, USA and an Assistant Professor at SDU, Denmark. Since 2015 he has been with the University of Padova, as an Associate Professor first and as a Professor of Computer Engineering since 2020. He held Adjunct Associate Professor positions at Brown University and SDU. In 2016 he was a research fellow of the Simons Institute for the Theory of Computing, University of California, Berkeley. His research interests include: algorithms for data mining and machine learning, big data analytics, and applications to molecular biology and medicine. He has given more than 40 invited talks at international research institutions. He has won the best paper award in RECOMB 2013 and at ECML-PKDD 2022, and the test of time award at RECOMB 2023. He has been a a PI, co-PI or key researcher in projects funded by the Ministry of Education, University and Research (MIUR) of Italy, University of Padova, the EU (Horizon2020), and the NSF (USA).
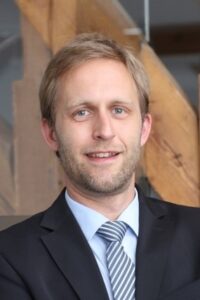
Björn Eskofier
Friedrich-Alexander-Universität Erlangen-Nürnberg
Head of Machine Learning and Data Analytics (MaD) Lab
Title
AI for Future Digital Healthcare: Towards Decentralized Care
Artificial Intelligence (AI) and Machine Learning (ML) methods are currently a “hot topic” in medicine. For instance, searching Pubmed publications for the keywords “Artificial Intelligence” or “Machine Learning” resulted in approximately 400 hits in 2000, then around 2200 in 2010, and then more than 21500 in 2020.
The driver of AI or ML method employment, in medicine as well as in other domains, is the availability of digital data. Despite the recent rise in publication numbers, the potential of delivering more objective, precise, and personalized medical diagnosis and care decisions is by far not reached. The reason is that the current healthcare data infrastructure nationally as well as internationally lacks interoperability and interfaces on several different levels (individual, institutional, device, and provider level, just to name a few). The health data infrastructure for future healthcare needs to address this, and one favored solution are “personal health dataspaces”, which put individuals into the center of data aggregation (figure), and creates new opportunities for AI and ML solutions for decentralized care. The talk will present the core idea of decentralized personal health dataspaces, and will hint at some opportunities for the future “digital” healthcare system that emerge from them. Concrete research projects that will be presented are concerned with the technical infrastructure for a personal health dataspace, and with contributing data to this dataspace from “digital health studies” (in immunology, neurology, women’s health, and others).
Bjoern M. Eskofier (SM, IEEE) heads the Machine Learning and Data Analytics (MaD) Lab at the Friedrich-Alexander-University Erlangen-Nuernberg (FAU). He is also the founding spokesperson of FAU’s Department Artificial Intelligence in Biomedical Engineering (AIBE), spokesperson of the German Ministry of Economic Affairs and Climate Action GAIA-X usecase project “TEAM-X” and co-director of the German Research Foundation collaborative research center “EmpkinS” (www.empkins.de). Since April 2023, he is an associate principal investigator and leader of the research group “Translational Digital Health” at the Helmholtz Zentrum Munich.
Dr. Eskofier studied Electrical Engineering at the FAU and graduated in 2006. He then did his PhD in Biomechanics under the supervision of Prof. Dr. Benno Nigg at the University of Calgary (Canada). He authored more than 400 peer reviewed articles, holds 5 patents, started three spinoff startup companies, and is in a supporting role for further startups. He won several medical-technical research awards, including the “Curious Minds” award 2021 in “Life Sciences” by Manager Magazin and Merck and the “Unipreneurs” award from the German Minster of Education and Research and the German Minister for Economy. In 2016, he was a visiting professor in Prof. Paolo Bonato’s Motion Analysis Lab at Harvard Medical School (February-March), in 2018, he was a visiting professor in Prof. Alex “Sandy” Pentland’s Human Dynamics group at MIT Media Lab (March-August), and in 2023 (April-August, he was a visiting professor in Prof. Scott Delp’s NMBL lab that is part of Stanford University’s Schools of Engineering and Medicine. He serves as Area Editor for the “IEEE Open Access Journal of Engineering in Medicine and Biology” and Associate Editor for the “IEEE Journal of Biomedical and Health Informatics”. He is also active in the organization of several IEEE and ACM meetings (e.g., BSN, BHI, EMBC, IJCAI, ISWC, UbiComp), most recently as General Chair of BHI (IEEE Biomedical and Health Informatics Conference) 2023.
Bjoern Eskofier has defined his research and entrepreneurial agenda to revolve around contributions to a “Digital Health Ecosystem”, where patients are connected to other stakeholders within the Healthcare system using digital support tools. His digital health research philosophy is that only multidisciplinary teams of engineers, medical experts, industry representatives and entrepreneurs will have the tools to actually implement changes in healthcare.

Davide Risso
University of Padova
Associate Professor of Statistics
Title
Statistical modeling of spatial transcriptomics data
Key biological processes depend on the physical proximity of cells and on the spatial organization of tissues. Furthermore, several diseases are characterized by abnormal spatial organization within tissues. In recent years technological advances have made it possible to quantify the expression of large numbers of genes while preserving the spatial context of tissues and cells. These techniques are collectively known as spatial transcriptomics (ST). Despite its early age, ST is rapidly becoming a widely used tool, complementing RNA sequencing to study gene expression in complex tissues, e.g., in cancer research and neurobiology. In addition to the gene expression measurements and the spatial localization of transcripts, available data include the raw images collected from the samples, that we can use to learn cell-level and tissue-level morphological features. Here, I will discuss some statistical and AI approaches to leverage ST as a diagnostic tool in the arsenal of pathologists in the era of personalized medicine and digital pathology.
Davide Risso is an Associate Professor of Statistics in the Department of Statistical Sciences of the University of Padova. received my Ph.D. in Statistics in 2012 from the University of Padua, He was a postdoctoral scholar at the Department of Statistics (2012 – 2014) and at the Division of Biostatistics (2014 – 2016) at the University of California, Berkeley. In the Fall of 2014 He was a Lecturer in the Division of Biostatistics, School of Public Health, University of California, Berkeley.
In February 2017 he joined the faculty of the Division of Biostatistics and Epidemiology of Weill Cornell Medicine as an Assistant Professor.
After being awarded a “Rita Levi Montalcini” fellowship, in 2018 He joined the Department of Statistical Sciences at the University of Padova as an Assistant Professor. His research focuses on developing, implementing, and applying statistical models and computational approaches for high-throughput biomedical data, including single-cell and spatial omics.
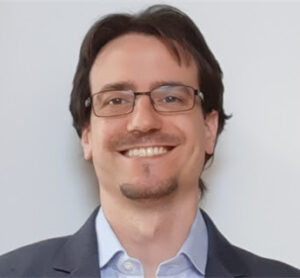
Mario Luca Morieri
Padova University
Researcher at Department of Medicine
Title
Unmet clinical need: the role of AI and clinical decision supporting systems
The healthcare landscape is marked by a persistent challenge: unmet clinical needs that hinder optimal patient care and outcomes. There is a global lack of appropriate implementation of guidelines’ recommendations in everyday clinical practice. For instance, in the cardiometabolic prevention field, real-world data shows a low achievement of recommended LDL-cholesterol targets or under-prescriptions of drugs with proven cardio-renal benefit. Numerous factors can contribute to these unmet needs, including, but not limited to, barriers within healthcare systems. This talk will discuss opportunities, gaps, and challenges presented by clinical decision support systems (CDSS) and artificial intelligence (AI) in addressing these clinical shortcomings. As medical complexities increase and healthcare data grows exponentially, CDSS can emerge as an important novel tool capable of augmenting clinical decision-making processes. Furthermore, the integration of CDSS with AI amplifies these potentials, fostering improvements in diagnostic accuracy, personalized treatment plans, and overall workflow efficiencies. While AI and CDSS are not yet applied in routine clinical practice, several studies and clinical trials are ongoing, and hopefully, they will pave the way toward novel approaches enhancing patient care.
Mario Luca Morieri, MD, PhD, currently serves as a Clinical Investigator at the University Hospital of Padova and his work revolves around precision medicine, utilizing genetics and real-world data to enhance care of patients with diabetes and dyslipidemia. He has been leading an innovative pharmacogenetic randomized clinical trial (MAGNETIC-PPARA, supported by the Italian Ministry of Health) to specifically test whether genetic variants can identify patients with better responses to cardiovascular preventive treatments, particularly fenofibrate. Dr. Morieri honed his expertise in cardiovascular and metabolic disease prevention graduating cum laude at the University of Bologna’s Medical School and completing his Internal Medicine residency at the University of Ferrara. Prompted by the interest in precision medicine, in 2015, he joined Dr. Alessandro Doria’s research group at the Joslin Diabetes Center (Genetics & Epidemiology Department) and Harvard Medical School in Boston, where he focused his research activities on identifying genetic markers for cardiovascular disease prediction and prevention in type 2 diabetes patients. Subsequently earned his PhD in Translational Medicine at the University of Padova. Here he engaged in real-world studies to enhance the translation of results from randomized clinical trials into everyday clinical practice, focusing on treatments with established cardiovascular benefits and addressing the disparities between daily clinical practice and guideline recommendations. Dr. Morieri has received numerous awards, grants, and fellowships, including recognition from the Italian Ministry of Health, Italian Society for the Study of Atherosclerosis, European Atherosclerosis Society, and American Diabetes Association. His scientific contributions are published in top-ranked journals such as NEJM, Diabetes, Diabetologia and Diabetes Care.
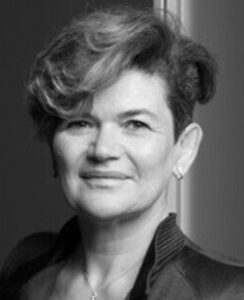
Giusella Finocchiaro
Bologna University
Full professor of Private Law
Title
AI and Regulation
Nowadays Artificial Intelligence is the most discussed topic in daily conversations: from useful applications in the healthcare to decision-making processes support and risks and dangers related to reality representation. Rules are frequently requested in order to govern the issue. But which rules? The need is for global rules for the regulation of a cross-border issue such as AI. But right now the main key player is European Union which is going to adopt a Regulation on Artificial Intelligence. May we talk about “Brussels effect” again? Will other legal systems be inspired by the AI Act? What is happening elsewhere in the world?
Giusella Finocchiaro is a Full professor of Private Law and Internet Law at the University of Bologna. She is a member of the Academy of Sciences of Bologna Institute. She is lawyer and has a legal boutique in Milan and Bologna, specialized in new technologies law, privacy, e-commerce and electronic signatures. She has been the Chairperson of the UNCITRAL Working Group on Electronic Commerce. She has been the Chairperson of the Commission established at the legislative office of the Ministry of Justice charged with drafting legislative decrees for updating national law in line with the European Regulation on personal data protection. She is a legal expert of the World Bank. She is an expert of UNIDROIT’s Digital Assets and Private Law Project.
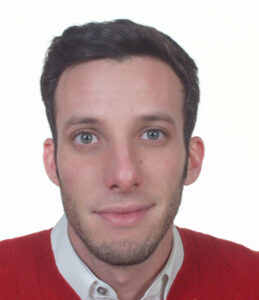
Alessandro Fabris
Max Planck Institute for Security and Privacy, Bochum
Research fellow
Title
More than an afterthought: don’t make AI fair, make fair AI
While artificial intelligence (AI) undergoes cycles of enthusiasm and stagnation, its growing success is undisputed. Accurate applications demonstrate AI’s utility across domains, making trustworthiness questions more pressing than ever. Medical algorithms developed without considering fairness will perform poorly for vulnerable groups and individuals, providing them with worse healthcare. In this talk, I present the main factors leading to undesirable algorithmic discrimination along with contextualized mitigation strategies to extend the benefits of AI to vulnerable populations.
Alessandro Fabris is a research fellow at the Max Planck Institute for Security and Privacy working on fairness and non-discrimination in algorithms. His research centers on defining, operationalizing, and measuring fairness, with a focus on domain-specific requirements and a critical perspective on data ethics. Previously, he spent four years in the industry with IBM and Electrolux. He works to guide and translate policy into responsible computing practices that practitioners can adopt.

Fabio Grigenti
Padova University
Department of Philosophy, Sociology, Education and Applied Psychology
Title
Risks and reliability. The European way in the ethical regulation of AI
Artificial intelligence (AI) has profoundly changed and will continue to change our lives. AI is being applied in more and more fields and scenarios such as autonomous driving, medical care, media, finance, industrial robots, and internet services. The widespread application of AI and its deep integration with the economy and society have improved efficiency and produced benefits. At the same time, it will inevitably impact the existing social order and raise ethical concerns. Ethical issues, such as privacy leakage, discrimination, unemployment, and security risks, brought about by AI systems have caused great trouble to people. Therefore AI ethics, which is a field related to the study of ethical issues in AI, has become not only an important research topic in academia, but also an important topic of common concern for individuals, organizations, countries, and society. My contribution will give a comprehensive overview of this field by summarizing and analyzing the ethical risks and issues raised by AI, ethical guidelines and principles issued by European context, approaches for addressing ethical issues in AI, methods for evaluating the ethics of AI.
Fabio Grigenti is full professor of History of Philosophy and Philosophy of Technology at the FISPPA (Department of Philosophy, Sociology, Pedagogy and Applied Psychology) of the University of Padua. He trained between Italy and Germany, obtaining a doctorate in philosophy with a dissertation on Schopenhauer and the philosophy of nature. Subsequently, he conducted research in philosophical historiography, history of science and philosophical anthropology. He has directed and participated in several international research projects and has held courses and lectures in European universities (TUDA – Darmstadt, Charles Prague, Munich, Freie Berlin) and South America (Cordoba and Florianopolis). He is the author of numerous scientific publications, in the last period mostly aimed at the History of the Philosophy of Technology (The machine and thought, Orthotes 2021) and the field of artificial intelligence. He edited the first Italian translation of Alan Turing’s essay The chemical bases of morphogenesis (Mimesis 2022).

Guglielmo Tamburrini
Federico II Napoli University
Professor of Philosophy of Science and Technology
Title
AI task autonomy and the ethics of medical supervision
In this short contribution, I will consider from an ethical viewpoint the increasing levels of autonomy that AI systems are achieving in a variety of task environments, especially focusing on medical domains. In particular, I will consider the challenge of fulfilling the duty of medical supervision in the light of the opacity of major AI deep learning systems. I will additionally examine the related challenge of managing informed consent and maintaining trust relationships between medical personnel and patients. Finally, I will illustrate possible tensions between the ethically motivated goal of preserving human supervision of AI systems and medical beneficence goals.
Guglielmo Tamburrini (PhD in Philosophy 1987, Columbia University) is Philosophy of Science and Technology Professor at Universita’ di Napoli Federico II in Italy. His current research interests focus on ELSE (Ethical, Legal, and SocioEconomic) issues arising in the context of Artificial Intelligence (AI), human-computer and human-robot interactions. Coordinator of the first European project on the ethics of robotics (ETHICBOTS, VI FP, 2005-2008). He was awarded the Giulio Preti International Prize in 2014 by the Regional Parliament of Tuscany for Toscana for contributions to the dialogue between Science, Philosophy and Democracy. Currently member of ICRAC (International Committee for Robot Arms Control) and scientific board member of USPID (Unione degli Scienziati per il Disarmo).
Carlo Combi
Verona University
Full Professor of Informatics
Title
Explainable AI in Medicine: towards a foundational definition
The rapid increase of interest in, and use of, artificial intelligence (AI) in computer applications has raised a parallel concern about its ability (or lack thereof) to provide understandable, or explainable, output to users. This concern is especially legitimate in biomedical contexts, where patient safety is of paramount importance. This talk tries to explore in some depth the concept of explainable AI, or XAI, offering a functional definition and conceptual framework or model that can be used when considering XAI. This is followed by a series of desiderata for attaining explainability in AI, each of which touches upon a key domain in biomedicine.
Carlo Combi was born in 1962. In 1987 he received the Laurea Degree in E.E. by the Politecnico of Milan. In 1993 he received the Ph.D. degree in biomedical engineering. He was in 1994 and 1995 Post-Doc fellow at the Department of Biomedical Engineering of the Politecnico of Milan. From 1987 to 1996 he worked within the research group in Medical Informatics at the Politecnico of Milan. From April 1996 to October 2001, Carlo Combi was with the Department of Mathematics and Computer Science of the University of Udine as Assistant Professor. Since November 2001, he is with the Department of Computer Science of the University of Verona. From November 2001 to February 2005, he was Associate Professor of Computer Science; since March 2005, he is Professor of Computer Science. From October 2007 to September 2012, he was head of the Department. From July 2009 to June 2013 he was chair of the Artificial Intelligence in Medicine Society (AIME). He is author, with Elpida Keravnou (University of Cyprus) and Yuval Shahar (Ben-Gurion University of the Negev) of the book Temporal Information Systems in Medicine, Springer, 2010.
Main research interests are related to the database field, with an emphasis on the management of clinical information. The two main topics are: temporal information systems and multimedia databases.
Panagiotis Papapetrou
Stockholm University
Professor and Vice Head of Computer and Systems Sciences
Title
Explainable and reliable AI for healthcare
Recent advances in the areas of artificial intelligence and machine learning and their application to knowledge discovery from medical data sources has been receiving increasing attention over the past several years. At the same time, the adoption of Electronic Health Records (EHRs) together with the availability of patient self-management and empowerment technologies give rise to new forms of health-related data sources of multi-modality and high complexity requiring novel data representation and analytics workflows. Particular challenges that arise include the representation of the involved complex feature spaces, dealing with data sparsity and missingness, exploiting the temporality nature of the data sources, and maintaining good trade-offs between model performance and explainability.
In this talk, I will focus on data variables of sequential nature related to heath. These variable types include spatial trajectories, panel data from longitudinal studies, time series signals (such as ECGs), event sequences (such as sequences containing EHR events), and mHealth data. I will emphasize the importance of mining and knowledge discovery from these data sources in the context of research questions posed by medical researchers and clinicians. I will further elaborate on how the integration and fusion of such data sources of heterogeneous nature can result in improved model performance in terms of predictive power, reliability, and scalability. Further, I will discuss and elaborate on the need for explainable machine learning models and their applicability in medical decision making problems that require trustworthy recommendations.
Panagiotis Papapetrou is a Professor at the Department of Computer and Systems Sciences of Stockholm University. His area of expertise is algorithmic data mining with particular focus on time series classification, explainability, and emphasis on healthcare applications. Panagiotis received his PhD in Computer Science at Boston University in 2009 and his Masters degree at the same university in 2007. He was a postdoctoral researcher at Aalto University during 2009-2012, and a lecturer at Birkbeck University of London, UK, during 2012-2013. He has participated in several national and international research projects, among which a 4-year starting grant funded by the Swedish Research Council. He is serving as Action Editor at the Data Mining and Knowledge Discovery journal and he is a Board Member of the Swedish Artificial Intelligence Society. Panagiotis has been involved in the organization of several Workshops and Tutorials at KDD, ICDM, and ECML/PKDD. Moreover, he has served as the general chair of IDA 2016 ans 2024, PhD consortium co-chair at ICDM 2018, Workshops co-chair at ICDM 2019, and journal track chair for ECML/PKDD 2024.

Pietro Lió
Dept. of Computer Science, Cambridge Center of AI and Medicine
Professor of Computational Biology
Title
Actionable and responsible AI in medicine
In this talk I will describe the AI Challenges in Medicine; then I will provide sme examples of using Graph relations as neural networks (GNN) from large dimensions (population) to small ones (precision, molecular):
- Predicting patient outcome and exploiting cohort information;
- Building the patient digital twin: exploiting information from different tissues, organs; complexing GNN with hypergraphs and sheaf. I will consider physiological (cardiovascular), clinical (inflammation) and molecular variables (multi omics and genetics)
- How to use GNN and diffusion models to design molecules.
Finally I will discuss methodologies that help keeping the clinicians in the loop to avoid excessive automatisation using logic and explainer frameworks.
Pietro Lió received the PhD degree in complex systems and non linear dynamics from the School of Informatics, dept of Engineering, University of Firenze, Italy and the PhD degree in Genomics from the University of Pavia, Italy. He is currently a professor of computational biology with the Department of Computer Science and Technology, University of Cambridge and a member of the Artificial Intelligence Group.
He is also a member of the Cambridge Centre for AI in medicine, ELLIS (European Laboratory for Learning and Intelligent Systems), Academia Europaea, Asia Pacific Artificial Intelligence Association, . His research interests include graph representation learning, AI and Medicine, Systems Biology.

Lorenzo Chiari
Bologna University
Full Professor of Biomedical Engineering
Title
The DARE initiative: a national flagship leveraging on AI and digital technologies for better health promotion and prevention
Artificial Intelligence is by far the most popular digital technology currently being used in the framework of the DARE initiative. DARE (2022-2026) is a national flagship funded by the Italian Ministry for University & Research (MUR) within the National Recovery and Resilience Plan Complementary Investments (PNC) on “Research initiatives for technologies and innovative trajectories in the health and care sectors”. Its primary focus is on health data valorization. Through more than 65 pilot projects ranging from primary to secondary and tertiary prevention, it is working to enhance the tools, knowledge, and processes (technical, ethical-legal, and organizational) that allow us to exploit the tremendous potential of data to define, monitor, and even predict health trajectories for the sake of health promotion and prevention with a lifelong perspective. This talk will present the latest achievements of the initiative, mainly focusing on community-based AI-driven primary prevention.
Lorenzo Chiari is a Professor of Biomedical Engineering at the University of Bologna. His research interests are digital health, particularly telerehabilitation and healthy and active aging, analysis of movement, balance, and fall risk with wearable sensors, and digital biomarkers discovery. He directed the Interdepartmental Center on Health Sciences and Technologies and is a member of the university research advisory board (GTA) on Horizon Europe’s Cluster 1, Mission Cancer, and Innovative Health Initiative. He was the PI of 3 European projects (SENSACTION-AAL, FARSEEING, CuPiD) on innovative technologies for Aging and Wellbeing and Personal Health Systems. Since December 2022, he has been President of the Board of Directors of the “DARE – Digital Lifelong Prevention” Foundation and PI of the research project of the same name. DARE (2022-2026) is funded by the Italian Ministry of University and Research and has the ambitious objective of establishing Italy as a leading country in digital prevention.
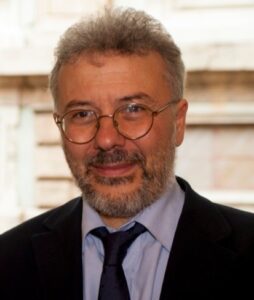
Michele Piana
Genova University
Full professor of Numerical Analysis
Title
Multi-scale computational modelling of oncological and neurological data
Oncological and neurological diseases can be described thanks to multi-modal information characterized by many different scales, ranging from genomics, through proteomics and cellular, to imaging data. This talk will describe how computational schemes based on inverse problems theory, pattern recognition, supervised and unsupervised machine learning can be used for the interpretation of neurophysiological times series, positron emission tomography volumes, genomics and proteomics data, to identify data-driven biomarkers and to design possible prototypes of bio-digital twins. Clinical applications will involve both solid and liquid cancers, and different types of neurodegenerative diseases.
Michele Piana is professor of numerical analysis at the Università di Genova, associate researcher at the IRCCS Ospedale Policlinico San Martino Genova, and associate researcher at the “Osservatorio Astrofisico di Torino, Istituto Nazionale di Astrofisica”. He is principal investigator of the MIDA group (hAps://mida.unige.it/) at the Università di Genova and director of the Life Science Computational Lab at the IRCCS Ospedale Policlinico San Martino Genova. He is Technical Director for DHEAL-COM, Piano Nazionale Complementare, Ministero della Salute, and co-Investigator for the ESA STIX mission. His research is focused on inverse problems, machine learning, and computational data analysis in medicine and astrophysics. Applications involve functional and morphological medical imaging, computational medicine, computational biology, high-energy solar physics, space weather. He is and has been principal investigator and work package leader in several national, EU, and international research projects and is involved in several collaborations under industrial contracts. He authored and co-authored one book on solar hard X-ray imaging, almost 200 refereed papers, 3 book chapters and more than 20 conference publications.
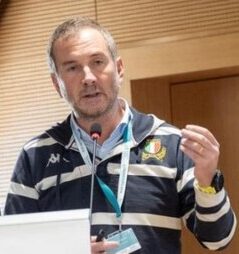
Dario Gregori
Padova University
Full professor of Medical Statistics
Title
Wearable devices in clinical research and prevention
Smartwatches and other wearable devices are popular tools for people to monitor and improve their health and well-being in their daily lives. These devices can collect and process large amounts of continuous data on behavioral or physiological functions, such as heart rate, blood pressure, sleep quality, and physical activity. By using big data and artificial intelligence techniques, these data can be analyzed to provide more accurate and personalized insights into the health status of the users, as well as to detect potential anomalies or risks. Wearable devices have many clinical applications, such as screening for arrhythmias in high-risk populations, managing chronic diseases such as heart failure or peripheral artery disease remotely, and even supporting oncology care by tracking symptoms, side effects, and quality of life of cancer patients.
DG is full professor of medical statistics at University of Padova. After graduation in Statistics at Pennsylvania State University (US) he got a PhD in Applied Statistics in 1995 at University of Firenze. He is Director of the residency program in Medical Statistics and Biometrics and Coordinator of the Ph.D. Program in Specialized and Translational Medicine “G.B.Morgagni” at University of Padova. His interests include clinical predictive modeling and machine learning algorithms for bio-medical research, including big data use for primary and secondary prevention. He holds several grants in this field from national and international agencies. He published more than 600 papers (H-index 48).
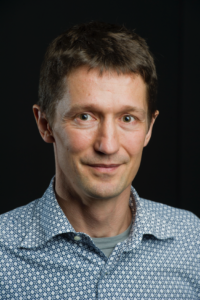
Henning Müller
HES-SO Valais & University of Geneva, Switzerland
Full Professor of Computer Science
Title
AI for medical image analysis: ExaMode and beyond
Machine learning can be used for all types of data and on image analysis the results have often been impressive. By combining text and images even better results can be achieved, particularly when looking at weak supervision for the training.
This presentation will detail several examples from the ExaMode project on multimodal data analysis and how to limit manual work required for the training. Applications in this case are on histopathology data but can easily be deployed to other fields on mediacl image analysis. The presentation will also give example on how explainable Ai can increase trust in algorithm outcomes.
Henning Müller studied medical informatics at the University of Heidelberg, Germany, then worked at Daimler-Benz research in Portland, OR, USA. From 1998-2002 he worked on his PhD degree in computer vision at the University of Geneva, Switzerland with a research stay at Monash University, Melbourne, Australia. Since 2002, Henning has been working for the medical informatics service at the University Hospital of Geneva. Since 2007, he has been a full professor at the HES-SO Valais and since 2011 he is responsible for the eHealth unit of the school. Since 2014, he is also professor at the medical faculty of the University of Geneva. In 2015/2016 he was on sabbatical at the Martinos Center, part of Harvard Medical School in Boston, MA, USA to focus on research activities. Henning is coordinator of the ExaMode EU project, was coordinator of the Khresmoi EU project, scientific coordinator of the VISCERAL EU project. Since early 2020 he is also a member of the Swiss National Research Council.
Filippo Lanubile
University of Bari
Head of Department of Informatics
Title
Machine Learning Development Operations (MLOps) for healthcare
Data-driven Artificial Intelligence (AI), such as Machine Learning (ML), is increasingly been experimented in healthcare. However, most of the attempts never go past the prototypical stage of ML model building. This occurs because constructing a model is just a small part in the process of creating production-ready ML-enabled systems that need to operate in a safety-critical and highly regulated domain such as healthcare. MLOps comes to help, being a set of practices and tools that combine machine learning and software engineering. In this talk, I will discuss how MLOps can be applied to bridge the gap between ML prototypes developed by data scientists and production systems developed by software engineers, enabling the continuous deployment of software components powered by ML, and assuring the quality of both data and models after being deployed online.
Filippo Lanubile is a Full Professor of computer science and Head of the Department of Informatics at the University of Bari, Italy, where he also leads the Collaborative Development Research Group. His research interests include: human factors in software engineering, AI-augmented software development, and software engineering for AI/ML systems. He is a recipient of a NASA Group Achievement Award, an IBM Eclipse Innovation Award, an IBM Faculty Award, and the Software Engineering Innovation Foundation Award from Microsoft Research. He was the General Chair of the 2020 and 2021 editions of the ACM/IEEE International Symposium on Empirical Software Engineering and Measurement (ESEM) and he is currently the Chair of the IEEE Software Advisory Board.
Barbara Di Camillo
Department of Information Engineering, Padova University
Full Professor of Computer Science
Title
In order to bring AI in real clinical settings, however, it is of paramount importance that Brainteaser tools are compliant with principles of trustworthy AI, including the assessment of the accuracy and generalizability of the results; the clarification of the domain of validity and the explainability of the predictions which in turn, ensure a human in command approach. During the talk, I will delve into these critical aspects, drawing insights from the practical experience gained through the implementation of BRAINTEASER.
Barbara Di Camillo received the master’s degree in electronic engineering from the University of Padova, in 2000, and the PhD degree (Dottorato di Ricerca) in biomedical engineering from the University of Padova, in 2004. She is full professor in computer science with the Department of Information Engineering, University of Padova. She was a visiting scientist with Mayo Clinic (Rochester, MN), “endocrinology research group” led by Dr. S. Nair, in 2002 and at the “Bioinformatics Center of University of Technology of Graz” (Graz, Austria), led by Prof. Trajanoski in 2003. Her research activity is centered in the development and application of advanced modeling, data mining and machine learning methods for high-throughput biological data analysis in the field of Bioinformatics and Systems Biology. In particular, she has developed and applied different methods for robust biomarker discovery, predictive modeling and clustering of next generation sequencing (NGS) data. She has also a great expertise in the development and application of differential equation based models, Boolean and Bayesian Networks for modeling of transcriptional networks and signaling pathways.

Tommaso Banzato
Padova University
Associate Professor
Title
AI in Veterinary Medicine: a potential bridge between Human and Veterinary Healthcare
The use of dogs as animal models of spontaneous pathology has the potential to provide valuable insights for the diagnosis and treatment of several human diseases. On the other hand, for a comparative interpretation of the data collected in dogs the differences and similarities on how dogs and people develop and respond to such diseases must be thoroughly explored. In this talk we will go through the canine pathologies that more closely resemble their human counterpart and will discuss how AI-based multimodal algorithms could unlock the potential of dogs as animal models of human diseases. Finally, this session will make a quick overview of the state of the art of AI in veterinary medicine.
Prof. Tommaso Banzato is an Associate Professor at the University of Padua. He obtained his DVM degree at the University of Padua and his PhD degree in 2013 at the same university with a thesis on the normal diagnostic imaging features of snakes, and lizards. After the PHD he had a post-doctoral position until 2018 when he became assistant professor at the same university. Prof. Banzato has authored more than 50 peer-reviewed research publications in diagnostic imaging. He performed an extensive research activity focused on the standardization of different imaging techniques in exotic pets including snakes, lizards, rats, rabbits, and birds. At present time his research is mainly focused on the possible applications of deep learning on diagnostic imaging of companion animals. He was recently funded by the University of Padua for a project aimed to use deep learning to predict the grading of human meningiomas using a comparative application of a methodology he has developed in dogs. Finally, Prof. Banzato was recently funded by the Italian Ministry of University and Research with a project focused on the comparative study of ageing in dogs and humans.

Marco Zorzi
Padova University
Full Professor at Department of General Psychology
Title
AI in Stroke Neuroimaging: From brain to behaviour
Artificial intelligence is increasingly used on neuroimaging data to predict clinical outcomes in neurological diseases. In the case of brain stroke survivors, AI can be used to predict patients’ post-stroke deficits and long-term functional recovery across behavioural domains that range from motor function to language and memory, with significant translational potential. However, data scarcity has forced the adoption of relatively simple machine learning methods, which have limitations but also strengths in relation to model explainability. I will discuss how machine learning has been applied to both structural and functional neuroimaging data, with predictive accuracy that varies across imaging methods and behavioural domains. I will then discuss how data scarcity can be addressed using data augmentation and transfer learning methods that make the problem suitable for a deep learning approach, thereby outperforming shallow machine learning on behavioural domains where prediction remains challenging. Efforts in assembling larger datasets for more stringent out-of-sample performance assessment will be a key to enabling AI-based predictive models to move from bench to bedside.
Marco Zorzi is a Full Professor at the University of Padova, where he teaches Artificial Intelligence and Cognitive Psychology. He is also Deputy Director of the Padova Neuroscience Center (PNC) and Senior Researcher at IRCCS San Camillo Hospital in Venice, focusing on artificial intelligence applied to neuroimaging and neurorehabilitation. After completing his doctoral and postdoctoral studies in Trieste, London (UCL), and Padova, he began his academic career as an assistant professor at San Raffaele University in Milan in 2000. In 2001, he joined the University of Padova as an associate professor and has held the position of full professor since 2006. His laboratory (Computational Cognitive Neuroscience Lab) is engaged in interdisciplinary research at the intersection of cognitive science, computer science, and neuroscience to investigate the computational foundations of human learning and cognition. His research has received numerous grants and awards, including a prestigious grant from the European Research Council (ERC) for frontier research on generative learning in neural networks. He is an elected Fellow of the Cognitive Science Society and has authored more than 170 articles in international journals.
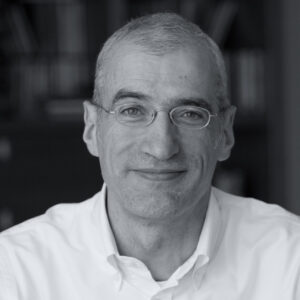
Alfonso Pozzan
Evotec Verona
Molecular Architect department Director
Title
AI and Machine Learning in drug discovery

Riccardo Bellazzi
Pavia University
Full Professor and Director of the Department of Electrical, Computer and Biomedical Engineering
Title
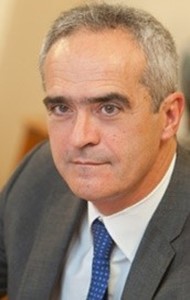
Rosario Rizzuto
Padova University
Full Professor of General Pathology
Title
From molecular elucidation of pathogenesis to personalized medicine: the opportunities of new biomedical technologies
Medicine faces today the formidable challenge of taking advantage of the continuous progress in the molecular understanding of diseases, and in the appreciation of the individual differences in pathogenesis, for developing highly effective “personalized” drugs in a sustainable manner. In other words, in order to develop better cures for human diseases, it will be necessary to develop a growing number of drugs tailored for the individual patients, without however exposing the health systems to the unsustainable costs of many drugs acting on smaller number of individuals.
To circumvent this conundrum, it will be crucial to exploit the key emerging technologies of Gene Therapy and RNA therapeutics through a multidisciplinary effort, encompassing molecular biology, genetics, biotechnology, pharmaceutics, pharmacology and clinical medicine. With this aim, the National Center for Gene Therapy and Drugs based on RNA Technology was created and funded by the National Recovery and Resilience Plan of the Italian University and Research Ministry (Mission 4, Component 2, Investment 1.4). Spearheaded by the University of Padua, the Center brings together 46 partners from the public, private and corporate sectors, spanning across Italy, coordinated to fulfill a 36-month research program. Specifically, research activity will be carried out by the participating partners within 10 thematic clusters (“spokes”) focused either on technology improvement (“horizontal spokes”) or on development of new therapies in the different disease areas (“vertical spokes”).
The strategic, overarching aim of the Center is to support efficacy and sustainability of the health system, in the framework of a competitive knowledge-based economy. The common purpose is to expand RNA-based pharmacology and gene therapy and develop new drugs for precision medicine. Further, the RNA Gene therapy National Center establishes cutting-edge physical infrastructures, and promotes educational and high-tech entrepreneurial initiatives.
Prof. Rizzuto is Professor of Pathophysiology at the School of Medicine of the University of Padua. He has been Rector of the University of Padua, and is currently President of the Foundation “National Center for Gene Therapy and Drugs with RNA Technology”, funded by the PNRR-MUR. Prof. Rizzuto has received several prizes, including the Chiara D’Onofrio Prize, the Biotec Prize, the Theodor Bucher Medal and the Antonio Feltrinelli Prize. He has organized international conferences and given >300 invited lectures at meetings and research institutions. He is a member of scientific academies (Academia Europaea, Accademia dei Lincei, Istituto Veneto di Scienze, Arti e Lettere, Accademia Galileiana) and of the European Molecular Biology Organization (EMBO). He acts as reviewer for numerous funding agencies, including ERC, and for major international journals (Nature, Science, Cell, etc.).
Prof. Rizzuto’s research is focused on cellular signals and in particular on the mechanisms and pathological alterations of mitochondrial homeostasis of calcium ions. His activity has been funded through the years by national and international agencies, such as ERC (Advanced Investigator Grant) and the EU Framework Programs, the Italian
Association for Cancer Research (AIRC), Telethon, the Ministries of Education and Health and the Cariparo and Cariplo Foundations. To date, he is the author of more than 330 publications in international journals cited by Pubmed (>50,000 citations, h-index 109, according to Scopus).

Martin Curley
Maynooth University, Innovation Value Institute
Director, Digital Health Ecosystem
Title
Radically transforming our health systems using digital, data and artificial intelligence
We have made remarkable progress as a global society with life expectancy more than doubling in just two hundred years through advances in public health, clinical care and pharmaceutical interventions. However, despite this amazing progress, modern healthcare systems are increasingly in crisis faced by spiralling costs and demands, aging populations and clinician attrition and morales issues. This presentation presents an optimistic view that our healthcare systems can be radically transformed, and life expectancy continue to be extended by three key interventions. The first intervention is a new paradigm and strategy for transforming our health systems called StayLeftShiftLeft-10X. The second intervention is the use of Open Innovation 2.0. a non-linear exponential change methodology co-developed by the European Commission, Intel and Maynooth University to drive a structural change in the health industry. The third intervention is the use of widespread complimentary artificial intelligence and data driven innovation solutions that enables a new kind of proactive, predictive, participatory and person healthcare system. In particular the presentation discusses why AI must be thought of in terms of Augment Intelligence to help avoid traditional adoption resistance. The presentation will give multiple examples of such solutions at work demonstrating benefits that are 10X (10 times) better than current solutions. Finally, the presentation will discuss why the decision to transform is as much a political decision as a clinical and technical decision and why we cannot delay in making this radical transformation decision.
Martin Curley is Professor of Innovation at Maynooth University and Director of the Digital Health Ecosystem at the Innovation Value Institute. Most recently Martin was Chief Information Officer and Director of Digital Transformation for Ireland’s National Health Service (HSE). Martin is chair of the UNGA Digital Health Symposium and has previously been Vice President and Global Direction of Digital Innovation at Intel Corporation and Senior Vice President at Mastercard and head of Global Digital Practice. Martin has been identified as a top 10 global influential, inspiring and impactful health leader/educator by a number of international publications and was previously European Chief Technology Officer of the year. He is author of eight books on Innovation, Digital and Entrepreneurship and keynoted and led executive education across all five continents.